Research on Object Navigation Method Based on Deep Reinforcement Learning (Working paper, in Chinese)
Jun 30, 2022·
·
0 min read

廖鸿浩
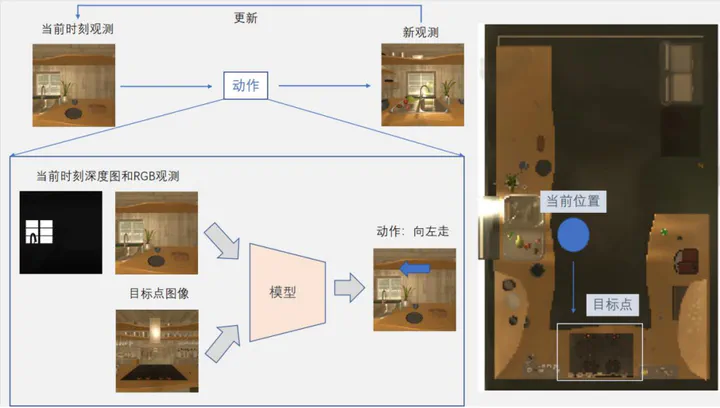
Abstract
Autonomous navigation is the basic ability requirement for robots to complete many other tasks. In recent years, the emerging deep reinforcement learning provides a solution for autonomous objective navigation of robots, but the existing visual objective navigation methods based on deep reinforcement learning have some problems such as poor cross-scene generalization ability. In this paper, an end-to-end visual object navigation model with strong ability of cross-scene generalization is proposed based on previous ideas. To solve the problem of weak cross-scene generalization, this paper proposes a state representation method combining objective detection results with depth image and a reward function representation method combining objective detection results. Since the state representation contains less scene-specific information, the combination of the state representation and the reward function representation ensures that the model can have strong cross-objective generalization ability as well as cross-scene generalization ability. In addition, the AI2THOR simulation scene is made into the Offline AI2THOR Dataset in this paper. Compared with the direct real-time rendering of AI2THOR simulation platform, the Offline AI2THOR Dataset can greatly improve the interaction speed and realize the cross-platform use of simulation scenes. On the basis of this dataset, the proposed navigation model is trained and experimented. Experimental results show that the proposed model has better navigation performance and has improved cross-objective generalization ability and cross-scene generalization ability.
Type